The integration of data science in the field of finance has brought about a significant transformation in the way financial institutions operate. By harnessing advanced analytical techniques and machine learning algorithms, data science has paved the way for exciting possibilities within the finance industry.
In this blog, we will explore how the revolutionary discipline of data science is reshaping and revolutionizing the world of finance. Knowing how vast the field of data science is, you need a well-structured approach to master it. Start acquiring knowledge by checking out the Data Science Course.
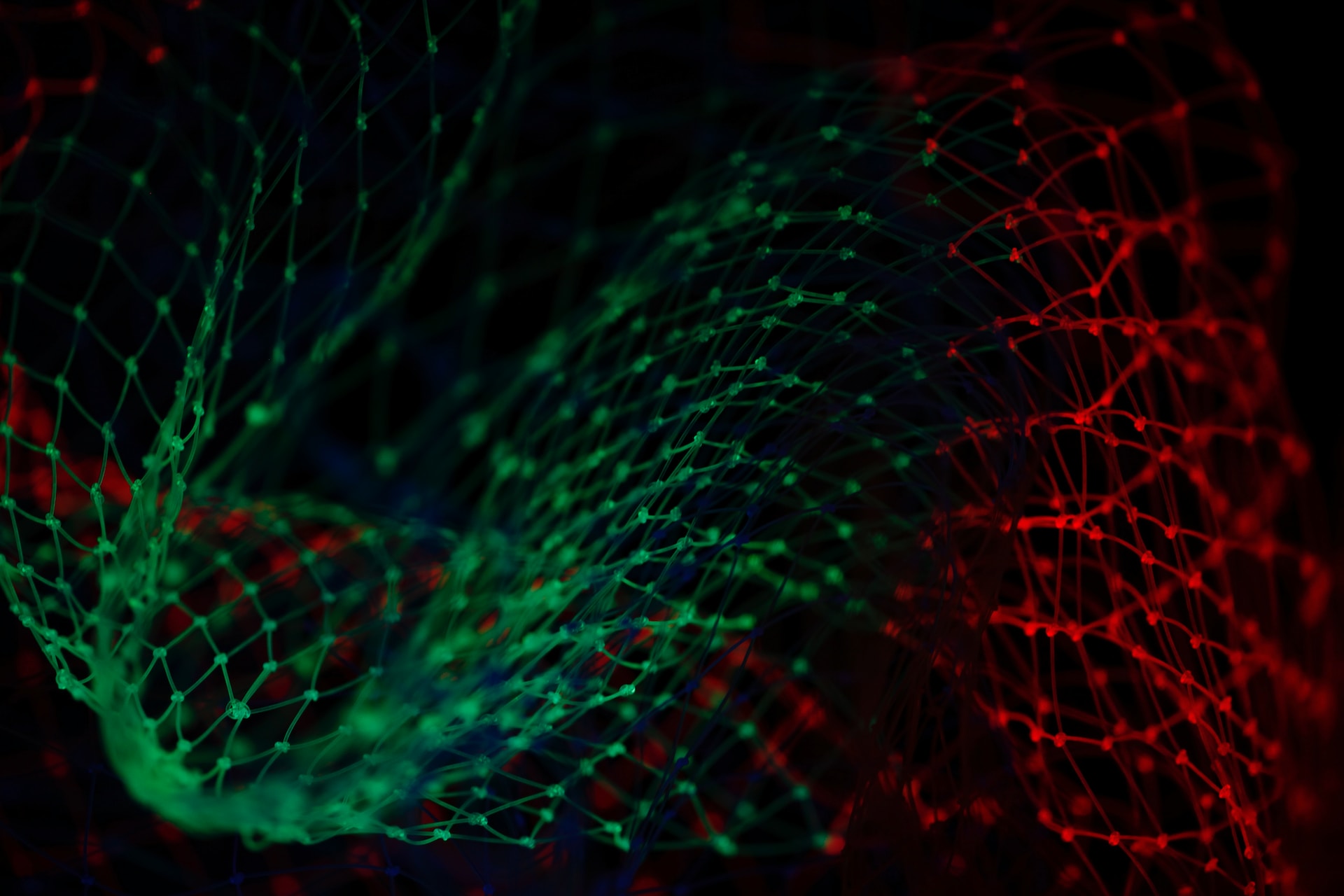
What is Data Science?
Data science encompasses the process of extracting valuable insights and knowledge from data, employing a combination of statistical analysis, machine learning techniques, and subject matter expertise. It encompasses data collection, preprocessing, analysis, modeling, and visualization to uncover patterns, trends, and actionable insights from vast amounts of data.
Data science plays a crucial role in transforming raw data into meaningful information that can drive strategic decision-making in various industries, including finance. The insights derived from data science empower organizations to optimize operations, mitigate risks, identify new opportunities, and ultimately gain a competitive edge in the dynamic world of finance.
Benefits of Data Science in Finance
There is multiple importance of leveraging data science in the financial sector, including:
- Empowered Decision-Making: By utilizing data science techniques, financial institutions can make well-informed decisions based on data-driven insights. Analyzing historical data and real-time market information allows them to identify trends, risks, and opportunities, leading to improved investment strategies and risk management practices.
- Enhanced Fraud Detection: Addressing the persistent issue of financial fraud, data science emerges as a powerful tool. By applying advanced analytics to detect anomalies and patterns, financial institutions can proactively identify and prevent fraudulent activities, safeguarding their customers’ assets.
- Personalized Customer Experience: Data science enables the analysis of customer data to gain deep insights into individual preferences and behaviors. This knowledge can be leveraged to tailor personalized financial products, recommendations, and services, resulting in heightened customer satisfaction and loyalty.
- Streamlined Operations: Through the implementation of data science, financial institutions can streamline their operations by automating manual tasks and optimizing processes. This boosts efficiency, reduces costs, and enables resources to be redirected toward strategic initiatives.
Applications of Data Science in Finance
The finance industry employs data science across various domains. Below are some of the applications of data science in the finance sector:
- Investment Analysis: By utilizing techniques like predictive modeling and sentiment analysis, data scientists can make accurate forecasts regarding stock prices, evaluate prevailing market trends, and fine-tune investment portfolios for optimal performance. These data-driven approaches provide valuable insights that empower investors to make well-informed decisions when it comes to their investments.
- Risk Assessment: Data science enables the development of sophisticated risk models to assess creditworthiness, market risks, and portfolio management. These models use historical data, market trends, and other factors to predict potential risks and inform decision-making.
- Algorithmic Trading: Data science techniques play a vital role in algorithmic trading, where algorithms analyze large datasets and execute trades based on predefined criteria. These algorithms help traders capitalize on market inefficiencies and make quick, data-driven investment decisions.
- Fraud Detection: Data science algorithms can detect patterns of fraudulent behavior by analyzing large volumes of transactional data. This enables early identification of potential fraud cases, protecting both financial institutions and customers.
- Customer Segmentation: Data science allows financial institutions to segment their customer base and identify high-value customers, enabling targeted marketing and personalized product offerings. Additionally, churn prediction models can help predict customer issues, allowing proactive measures to retain valuable customers.
Examples of Data Science in Finance
Let’s delve deeper into real-world examples of how data science is applied in finance.
- a) Credit Scoring: Data science models analyze historical data to assess creditworthiness and assign credit scores to individuals and businesses. These scores aid in making informed lending decisions, ensuring responsible and risk-conscious lending practices.
- b) Portfolio Optimization: Data science techniques optimize investment portfolios by analyzing historical market data, risk tolerance, and investment goals. These models help investors diversify their portfolios and maximize returns while managing risks effectively.
- c) Sentiment Analysis: Social media and news data can be analyzed using natural language processing techniques to gauge public sentiment towards specific financial assets or companies. This sentiment analysis can provide insights into market trends, investor sentiment, and potential impacts on stock prices, helping traders and investors make more informed decisions.
- e) Financial Forecasting: Data science models can leverage historical financial data, market trends, and economic indicators to forecast future financial performance. These forecasts assist in budgeting, financial planning, and strategic decision-making, providing insights into potential risks and opportunities.
Challenges and Limitations of Data Science in Finance
While data science has immense potential in finance, there are certain challenges and limitations to be aware of. It includes:
- Data quality and availability: Financial data can be complex, unstructured, and scattered across various sources. Ensuring data quality and accessibility for analysis can be a significant challenge.
- Privacy and security concerns: Finance deals with sensitive information, and data scientists must adhere to strict privacy and security regulations and financial industry compliance requirements. Handling and protecting confidential data can be challenging.
- Limited historical data: In finance, historical data plays a crucial role in making predictions and modeling risk. However, financial markets are dynamic, and historical data may not adequately capture future market conditions or unexpected events, limiting the accuracy and reliability of predictions.
- Regulatory and ethical considerations: Finance is heavily regulated, and data scientists must comply with laws and regulations related to data usage, privacy, and fairness. Balancing innovation with regulatory compliance and ethical considerations poses a challenge in developing data science solutions.
- Human expertise and domain knowledge: While data science brings powerful analytical capabilities, it still requires domain expertise and an understanding of financial markets, products, and regulations. Integrating human expertise with data-driven insights is crucial for effective decision-making.
- Rapidly evolving technology landscape: Technology advancements and new data sources constantly emerge in finance. Data scientists need to keep up with evolving tools, techniques, and emerging trends to leverage the latest technology effectively.
- Model validation and risk management: Validating and monitoring data science models is crucial in finance to assess their accuracy, reliability, and performance. Implementing robust risk management practices is essential to identify and mitigate potential model risks and failures.
Conclusion
In a world where numbers speak louder than words, Data Science has emerged as the ultimate ally for the finance industry. Data Science has revolutionized the field of finance, driving innovation and transforming the way financial institutions operate.
However, it’s important to acknowledge that while Data Science offers tremendous potential, it also presents certain limitations and ethical considerations. To fully unlock the benefits of Data Science in finance, professionals need to understand financial principles and data analysis techniques. As we move forward, the intersection of Data Science and finance will continue to evolve and shape the industry, so you should adhere to a well-constructed Data Science Training video consistently if you want to master data science.